Elevating Data Insights: Harnessing the Power of AWS and Databricks for Unparalleled Analytics
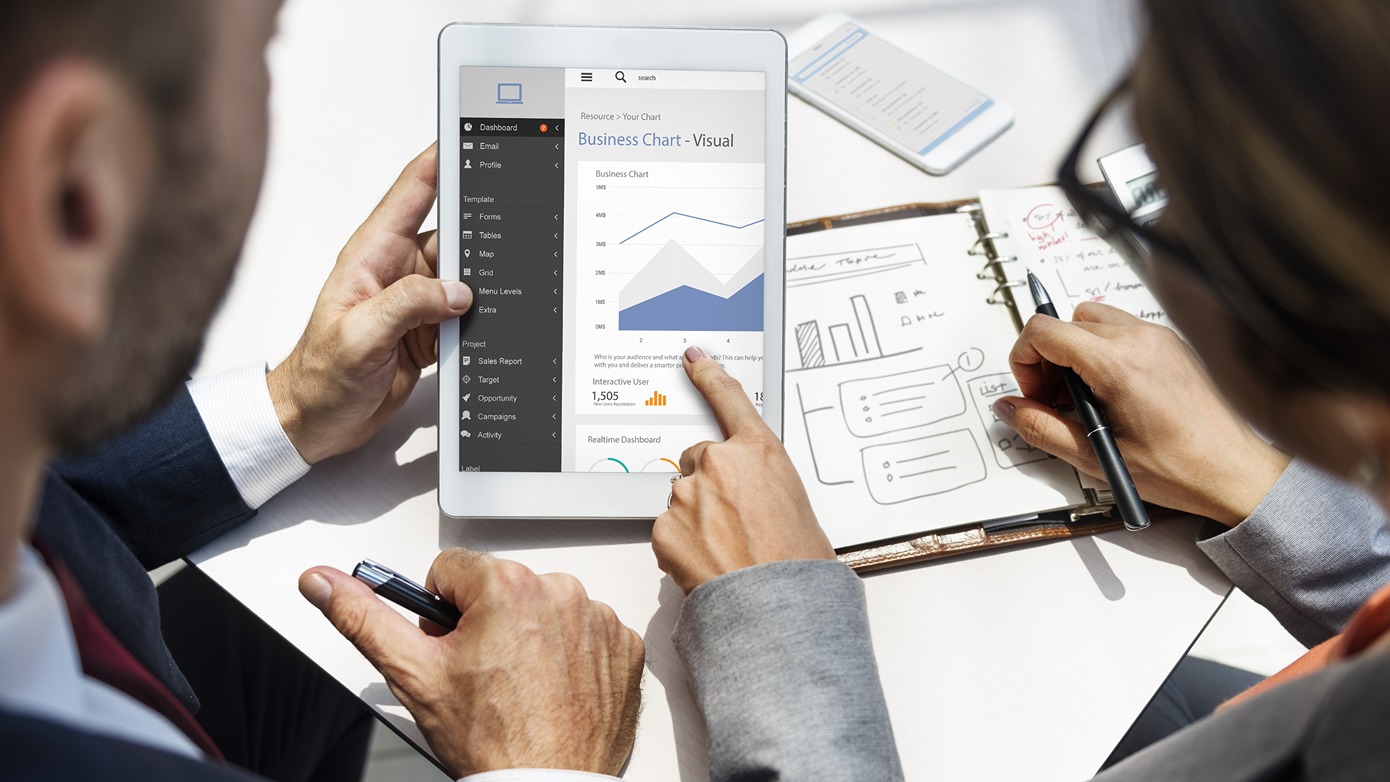
In today's data-driven world, organizations are constantly seeking innovative solutions to process, analyze, and derive valuable insights from vast amounts of data. Amazon Web Services (AWS) has emerged as a leading cloud platform, offering a plethora of services for scalable computing, storage, and data analytics. When combined with Databricks, an industry-leading unified analytics platform, businesses can unlock the full potential of their data and accelerate their journey towards data-driven decision-making. In this blog post, we will explore the benefits and implementation of AWS with Databricks to unleash the true power of data analytics.
Introduction to AWS and Databricks
Amazon Web Services (AWS) is a comprehensive and highly flexible cloud computing platform that offers a wide array of services, including computing power, storage options, and databases. AWS is renowned for its robustness, scalability, and cost-effectiveness, making it an ideal choice for organizations of all sizes.
Databricks, on the other hand, is a unified analytics platform built on Apache Spark. It simplifies big data and machine learning workflows by combining data engineering, data science, and business analytics into a collaborative workspace. Databricks allows teams to work together seamlessly and offers interactive querying, real-time streaming, and machine learning capabilities, making it a preferred platform for modern data analytics.
Why AWS with Databricks?
Integrating AWS with Databricks offers numerous advantages:
1. Scalability: AWS provides virtually unlimited resources, allowing Databricks to handle large-scale data processing and analytics. As data volumes grow, the system can effortlessly scale up or down, ensuring high performance and availability.
2. Cost-effectiveness: AWS's pay-as-you-go model and Databricks' optimized resource allocation help organizations manage costs efficiently. The ability to scale resources based on actual usage ensures that businesses pay only for what they consume.
3. Easy Setup: Deploying Databricks on AWS is a straightforward process. AWS's infrastructure-as-code capabilities, along with Databricks' user-friendly interface, enable organizations to set up a data analytics environment quickly.
4. Integration with Other AWS Services: Databricks seamlessly integrates with other AWS services such as Amazon S3, Amazon Redshift, and Amazon EMR, allowing users to leverage existing data storage and processing solutions.
5. Security and Compliance: AWS offers robust security features and compliance certifications, ensuring that data and analytics processes adhere to industry standards and regulations. Databricks also provides data encryption, access controls, and auditing capabilities to further enhance security.
Implementing AWS with Databricks
Let's walk through the key steps to implement AWS with Databricks:
Step 1: Setting up an AWS Account
To begin, create an AWS account or utilize an existing one. Once set up, navigate to the AWS Management Console to access the various AWS services needed for your data analytics workflow.
Step 2: Creating an S3 Bucket
An Amazon S3 bucket will serve as a data lake to store raw and processed data. Create a new bucket and configure access controls as per your requirements.
Step 3: Launching a Databricks Workspace
Go to the Databricks website and sign up for a workspace. The workspace acts as a collaborative environment for data engineers, data scientists, and business analysts to work together on data projects.
Step 4: Integrating Databricks with AWS
In the Databricks workspace, connect to your AWS account by providing the necessary credentials. This integration allows Databricks to access data stored in S3 and leverage other AWS services.
Step 5: Data Ingestion
Use Databricks to ingest data from various sources like databases, streaming platforms, or existing data stored in S3. The platform supports multiple data formats and provides easy-to-use APIs for data manipulation.
Step 6: Data Transformation and Analysis
Leverage the power of Apache Spark through Databricks to transform, clean, and process the ingested data. Perform exploratory data analysis, run complex queries, and gain valuable insights from your data.
Step 7: Machine Learning and Advanced Analytics
Utilize Databricks' machine learning capabilities to build and deploy models that can predict outcomes, classify data, or perform other advanced analytics tasks. The platform supports popular machine learning libraries and frameworks, making it suitable for data science projects.
Step 8: Visualization and Reporting
Create interactive data visualizations and dashboards in Databricks to communicate insights effectively to stakeholders. Databricks integrates with popular BI tools, allowing users to generate reports and share findings with ease.
Conclusion
AWS with Databricks is a powerful combination that empowers organizations to harness the full potential of their data. The scalability, cost-effectiveness, and seamless integration of AWS with Databricks make it a preferred choice for modern data analytics projects. By following the implementation steps outlined in this blog, businesses can accelerate their data-driven journey and stay ahead in today's competitive landscape. Whether it's data exploration, real-time analytics, or machine learning, AWS with Databricks can turn data into a strategic asset for informed decision-making.
Schedule a call with RevStar Consulting to get a free consultation.

Read On
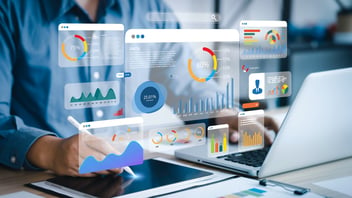
Efficient Data Processing with Databricks: Revolutionizing Big Data Analytics
In today's data-driven world, organizations are grappling with massive amounts of data generated at...
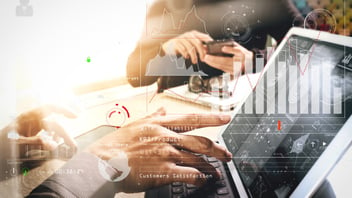
Scalability and Efficiency: Mastering Data Analytics with Databricks and Snowflake
Welcome to the data-driven revolution, where the twin forces of scalability and efficiency are...
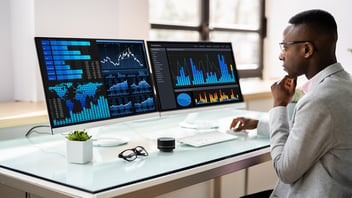
Scalability & Efficiency: Data Analytics with Databricks & Snowflake
In the vast landscape of modern business, data is a dual-faced entity – a challenge and an...
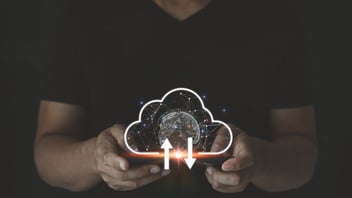
Data Migration: Ensuring Data Integrity and Security during the Transition
Data has emerged as a pivotal asset in the rapidly evolving landscape of technology and business....