Exploring Snowflake's Machine Learning Capabilities on AWS
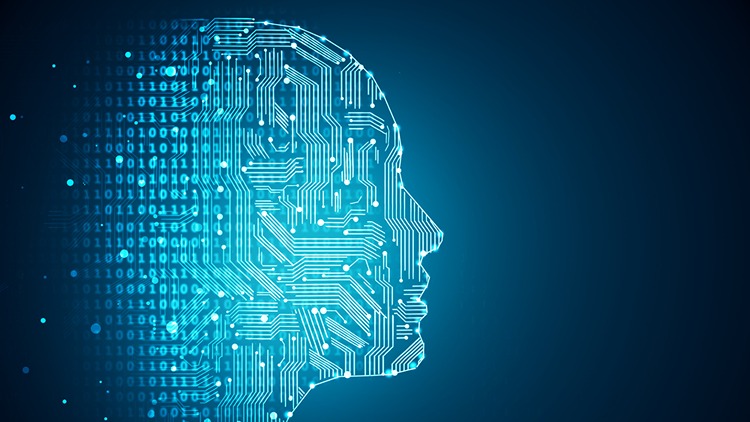
In today's data-driven world, organizations are constantly seeking innovative solutions to extract valuable insights from their vast amounts of data. Snowflake, a cloud-based data platform, has emerged as a leader in enabling seamless data warehousing and analytics. With its integration with AWS (Amazon Web Services), Snowflake offers a powerful combination of data storage, processing, and machine learning capabilities. In this blog post, we will delve into Snowflake's machine-learning capabilities on AWS and explore how it can empower businesses to unlock the full potential of their data.
Snowflake and AWS Integration
Snowflake's integration with AWS provides businesses with a scalable and flexible environment to leverage machine learning algorithms. By leveraging AWS's powerful infrastructure, Snowflake enables seamless data processing and model training, allowing organizations to focus on deriving meaningful insights rather than managing complex infrastructure.
Machine Learning Capabilities in Snowflake
Snowflake offers an array of machine-learning capabilities that can be seamlessly integrated into data workflows. These capabilities include:
a. Snowflake Data Science
Snowflake's Data Science capabilities allow data scientists to build, deploy, and manage machine learning models directly within the Snowflake platform. By leveraging familiar programming languages such as Python and R, data scientists can harness Snowflake's scalable compute resources to train models on large datasets and generate predictions and insights in real time.
b. External Model Integration
Snowflake's External Model Integration feature allows businesses to integrate machine learning models built using popular frameworks such as TensorFlow, PyTorch, or sci-kit-learn. By deploying these models directly within Snowflake, organizations can leverage the power of distributed computing to generate predictions at scale and gain actionable insights.
c. Data Pipelines with ML
Snowflake's data pipelines enable the seamless integration of machine learning workflows into data processing pipelines. Organizations can automate complex data tasks and generate real-time predictions and recommendations by combining Snowflake's data transformation capabilities with machine learning algorithms.
Integration with AWS Machine Learning Services
Snowflake's integration with AWS also extends to AWS's machine learning services, allowing businesses to leverage the full breadth of AWS's AI and ML capabilities. By seamlessly connecting Snowflake with AWS services such as Amazon SageMaker, Amazon Comprehend, and Amazon Rekognition, organizations can further enhance their machine-learning workflows and tap into advanced capabilities such as natural language processing, computer vision, and more.
Scalability and Performance
One of the key advantages of Snowflake's machine learning capabilities on AWS is its scalability and performance. Snowflake's cloud-native architecture allows for seamless scaling of compute resources based on workload demands. This means that organizations can train and deploy machine learning models on massive datasets without worrying about infrastructure constraints. Additionally, Snowflake's optimized query execution engine ensures lightning-fast performance, enabling businesses to generate insights in near real time.
Data Governance and Security
Snowflake strongly emphasizes data governance and security, which are paramount when dealing with sensitive data and machine learning models. Snowflake's platform offers robust security features such as data encryption, access controls, and auditing capabilities. By leveraging AWS's extensive security framework, organizations can ensure the confidentiality, integrity, and availability of their data throughout the machine learning process.
In conjunction with AWS, Snowflake's machine-learning capabilities provide organizations with a powerful platform to extract insights from their data at scale. By seamlessly integrating machine learning workflows into their data pipelines, businesses can unlock the full potential of their data and drive data-driven decision-making. Snowflake's scalability, performance, and emphasis on data governance make it a compelling choice for organizations looking to leverage machine learning in the cloud.
As the demand for machine learning continues to grow, Snowflake's integration with AWS positions it as a leader in the field, enabling businesses to stay at the forefront of data-driven innovation. By harnessing the power of Snowflake's machine learning capabilities on AWS, organizations can gain a competitive edge and drive impactful outcomes from their data assets.
Schedule a call with RevStar Consulting to get a free consultation.

Read On
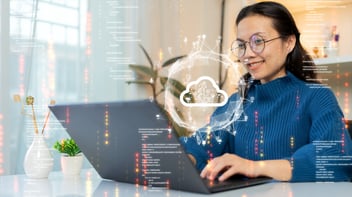
Scaling with Confidence: The CEO’s Guide to Growth with Snowflake’s Data Cloud
Data is the currency of success. For CEOs steering their companies through data-driven markets, the...
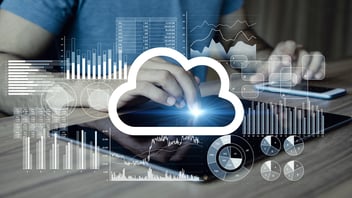
Snowflake and AWS: Top Architectural Considerations for High-Performance Analytics
Snowflake and Amazon Web Services (AWS) are two powerful players that, when combined, can take your...
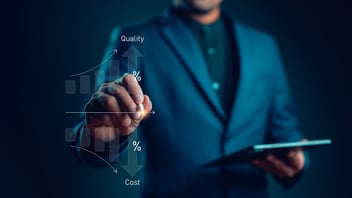
Optimizing Costs & Efficiency: The COO’s Roadmap to Integrating Snowflake and Databricks
Chief Operating Officers (COOs) play a critical role in driving efficiency and cost optimization...
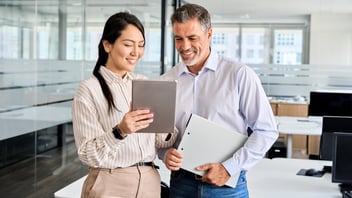
The Top 3 Challenges of Implementing Snowflake on AWS (and How to Overcome Them)
The implementation of Snowflake, the cloud data warehouse, on AWS can unlock tremendous value for...